Alexander von Rohr
Learning Systems and Robotics Lab, TU Munich.
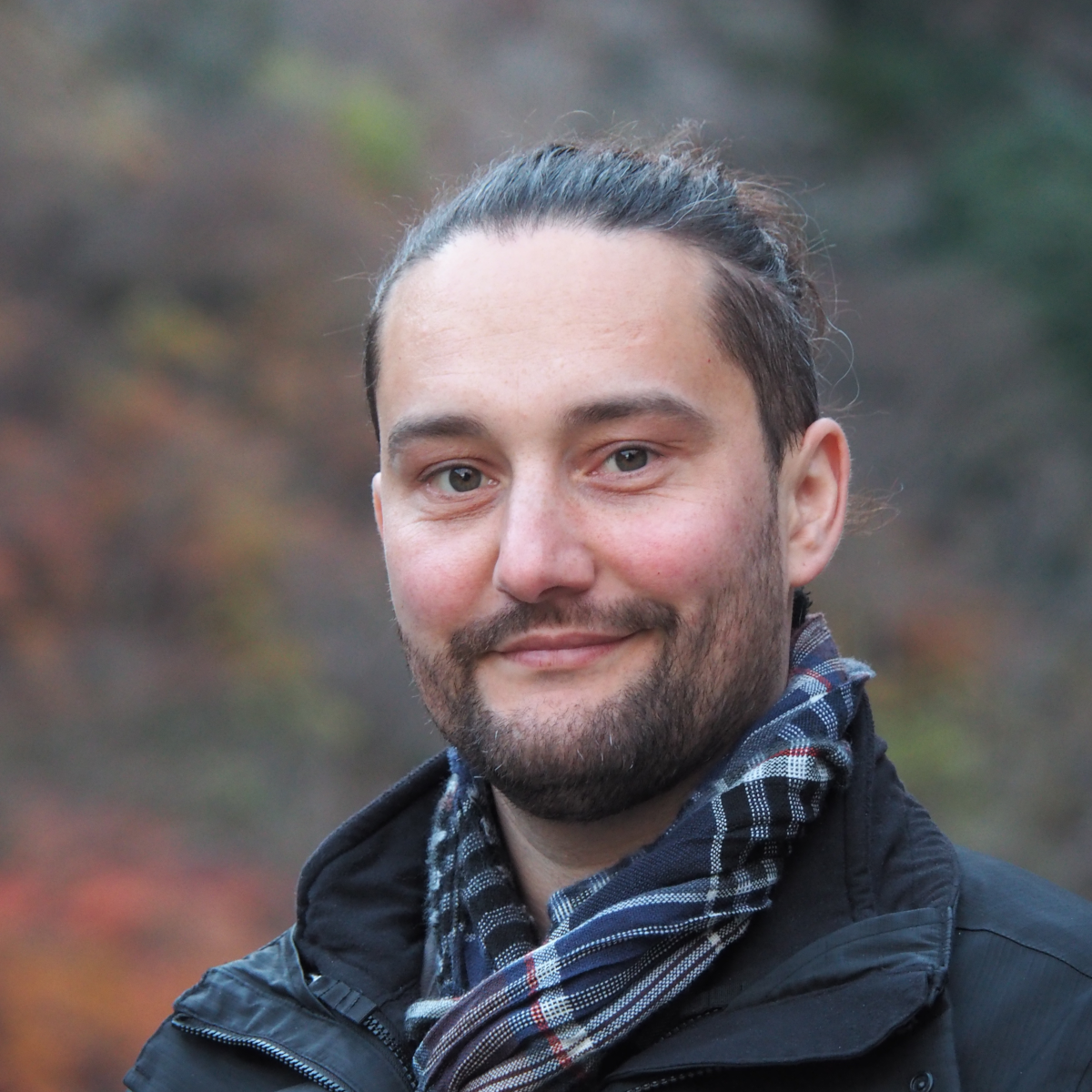
Building: N4, Room: A 2.11
Theresienstraße 90
80333 Munich, Germany
I am a postdoctoral researcher at the Technical University of Munich affiliated with the Learning Systems and Robotics Lab. My research is on embodied AI, specifically Bayesian optimization for robot learning, as well as risk-aware and robust reinforcement learning.
I conducted my PhD research at the Max Planck Institute for Intelligent Systems as a member of the Intelligent Control Systems Group and Institute for Data Science in Mechanical Engineering at the RWTH Aachen University, both led by Prof. Sebastian Trimpe. I was an associated scholar with the International Max Planck Research School for Intelligent Systems (IMPRS-IS), and my PhD was supported by IAV.
Before joining the Max Planck Institute, I studied Computer Science at the University of Lübeck. I earned my Bachelor’s degree in Electrical Engineering from BHT Berlin. Between these degrees, I worked as a full-time Software Engineer in Hamburg.
For a list of my research papers, see my Publications page. More information about my teaching activities is available here.
news
I received a Best Reviewer Award for my reviews at AISTATS 2025. | |
Our paper on Diffusion Predictive Control with Constraints got accepted at the 7th Annual Learning for Dynamics & Control Conference (L4DC). | |
I presented our work on Viability of Future Actions: Robust Reinforcement Learning via Entropy Regularization at the second mini-Workshop on Reinforcement Learning in Mannheim. | |
Our work on Simulation-Aided Policy Tuning for Black-Box Robot Learning has been published in the IEEE Transactions on Robotics (T-RO) and is now available in early access. Our new approach combines real-world & simulated data to fine-tune robot skills efficiently. See it in action: https://youtu.be/feMKxdnGXL4. | |
Pleased to announce that our latest paper, Event-Triggered Time-Varying Bayesian Optimization, is published in the Transactions on Machine Learning Research. This work introduces ET-GP-UCB, an algorithm that adaptively resets its optimization process in response to changes in a time-varying objective function, achieving efficient performance without prior knowledge of change rates. |